Pietsch, Wolfgang
(2014)
Aspects of theory-ladenness in data-intensive science.
In: UNSPECIFIED.
![[img]](https://philsci-archive.pitt.edu/style/images/fileicons/application_pdf.png) 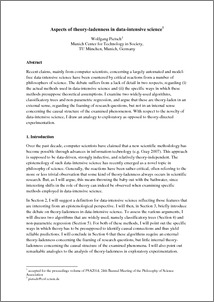 Preview |
|
PDF
pietsch_data-intensive-science_psa.pdf
- Accepted Version
Download (475kB)
|
Abstract
Recent claims, mainly from computer scientists, concerning a largely automated and model-free data-intensive science have been countered by critical reactions from a number of philosophers of science. The debate suffers from a lack of detail in two respects, regarding (i) the actual methods used in data-intensive science and (ii) the specific ways in which these methods presuppose theoretical assumptions. I examine two widely-used algorithms, classificatory trees and non-parametric regression, and argue that these are theory-laden in an external sense, regarding the framing of research questions, but not in an internal sense concerning the causal structure of the examined phenomenon. With respect to the novelty of data-intensive science, I draw an analogy to exploratory as opposed to theory-directed experimentation.
Monthly Views for the past 3 years
Monthly Downloads for the past 3 years
Plum Analytics
Actions (login required)
 |
View Item |