Zhang, Jiji and Spirtes, Peter
(2007)
Detection of Unfaithfulness and Robust Causal Inference.
In: UNSPECIFIED.
![[img]](https://philsci-archive.pitt.edu/style/images/fileicons/application_pdf.png) 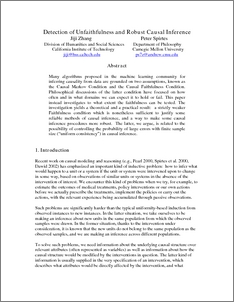 Preview |
|
PDF
Detection_of_Unfaithfulness_and_Robust_Causal_Inference.pdf
Download (179kB)
|
Abstract
Many algorithms proposed in the machine learning community for inferring causality from data are grounded on two assumptions, known as the Causal Markov Condition and the Causal Faithfulness Condition. Philosophical discussions of the latter condition have focused on how often and in what domains we can expect it to hold or fail. This paper instead investigates to what extent the faithfulness can be tested. The investigation yields a theoretical and a practical result: a strictly weaker Faithfulness condition which is nonetheless sufficient to justify some reliable methods of causal inference, and a way to make some causal inference procedures more robust. The latter, we argue, is related to the possibility of controlling the probability of large errors with finite sample size (“uniform consistency”) in causal inference.
Monthly Views for the past 3 years
Monthly Downloads for the past 3 years
Plum Analytics
Actions (login required)
 |
View Item |